- Rameez's Newsletter
- Posts
- The CMO's next big battle: over-attribution
The CMO's next big battle: over-attribution
Spoiler alert: this goes so, so much deeper than just the CMO...
Rameez Tase is the Co-founder & President of Antenna, a leading provider of advanced data analytics for subscription businesses. Before Antenna, Rameez served in pivotal roles as VP of Growth at Axios and Mic.
Whit Harwood works at MediaLink in Growth Strategy across tech, media & data sectors. Prior to MediaLink, Whit led Strategy & Growth teams at NBCU & multiple early stage startups.
In this post, Whit & Rameez team up to discuss some of the latest trends in marketing, including Retail Media Networks and marketing attribution (i.e. which half of my advertising spend isn’t wasted). The below analysis is purely personal opinion and does not represent the points of view of either Whit or Rameez’s employers.
AdExchanger’s James Hercher wrote an extremely prescient article last week: Walled Garden Platforms Are Drowning Marketers In Self-Attributed Sales. In it, he describes a looming problem that has huge consequences. The problem? Over-attribution: ad platforms claiming credit for driving a sale that they didn’t actually cause.
The problem: a single sale, a dozen claims
Here’s the negative feedback loop, from a CMO’s perspective:
Most large ad platforms are now Walled Gardens. A Walled Garden sells the ad, shows the ad on its own ad inventory, and measures the return on ad sales (‘ROAS’).
This is OK when 100% of a brand's advertising goes through a single channel, say, Instagram. If they advertise on Instagram, and someone who sees the ad also purchases the product that month — OK, Instagram probably had something to do with it.
But as more & more large, Walled Gardens emerge — Facebook, Google, Amazon, TikTok, Snap, Walmart, every other emerging Retail Media Network — this is a *huge* problem for brands. Why?
Because here's the view from the CMO's seat: if I show ads not only on Instagram, but also on a dozen platforms, and all of them are charging me based on the correlation between "saw the ad" and "bought the product," I'll end up paying up to 12x the actual value of the advertising. You can see how this will quickly go south for brands…
To exacerbate this problem, most of these large ad platforms have offsite audience networks: audience network ads are shown to people on unrelated websites (i.e. the Instagram audience network consists of websites unrelated to Instagram).
Why does this matter, in this context? Attribution is primarily correlation-based, rather than causation-based, increasing the chance that a person sees an ad via an audience network extension, regardless of whether it caused purchase. This is a highly strategic maneuver by Walled Gardens (and, conversely, the CMO’s nightmare).
The bottom line: As marketing channels enter a period of fragmentation (gone are the days of the Instagram-only marketing strategy), the number of Walled Gardens increase, and their walls get higher, CMOs will need an answer to ensure they don’t give away their entire margin to over-attributed advertising.
And, to be clear, this isn’t just the CMO’s problem; it’s the CFO’s, and the CEO’s as well. Consumer brands are built on the proposition that they can build, market, and distribute products & services more cheaply than they can charge for them. If, all of a sudden, the cost of marketing climbs precipitously without the corresponding increase to the number of people buying the product, nor the ability to charge more…

The solution: from correlation to causation
Put simply, a brand needs to be able to plainly see:
Ad spend data per channel
Sales data (as a result of that ad spend) per channel
The impact of that ad spend on specific audience segments across every platform
Requirement #1 is easy, every channel gives you that. Requirement #2 can be tricky, at times, especially in categories (e.g. retail) where brands often don’t have a direct-to-consumer relationship with a majority of their customers — more on that soon. But requirement #3 is a tough one. In a world of more channels + more Walled Gardens, a brands’ ability to understand where the same user was exposed, across their marketing portfolio, is critical.
The problem: there’s no unique identifier for brands to say “person 12345 saw an ad on this platform and not that one.” This isn’t the open web, and there’s no LiveRamp ID. In the absence of deterministic attribution, the preferred approaches are probabilistic Marketing Mix Modeling (“MMM”) and A/B testing (e.g. using geo holdout methodology) to estimate attribution.
In other words, if you can find shared attributes of a cohort that behaves similarly, and those attributes exist across each of your marketing channels, then you can loosely track that cohort across your entire portfolio. Or, you can simply test into it with a series of A/B/n tests.
There has been plenty of great content written on probabilistic attribution methods; so today, we’ll focus on three methods of data collection. How are CMOs aggregating all of the ad spend and sales data from these disparate Walled Gardens?
Method #1: The brand shares data with a single measurement partner
Companies like Measured and Kochava have shown success in their respective categories. Both companies connect (via API) into a brand’s marketing data, so they can collect information on ad spend; as well as their sales data, so they can calculate ROAS. These companies have provided immense utility to the brands they serve. Measured seems to be strongest amongst DNVB’s such as Vuori, while Kochava has found a home specifically for mobile advertising.
A single measurement partner with visibility across all marketing channels adds convenience: all your spend and sales data is one place. Once it’s all in the same place, running probabilistic attribution is easier — both aforementioned companies offer MMM and A/B testing. This works well for purely digital ad channels where the data is readily available via API.
Yes, but: Connecting to various third party ad platforms is great for convenience, and it does enable probabilistic attribution, but it doesn’t quite solve the canonical problem: without the ability to identify that a given person was exposed to advertising on each of those platforms, determining attribution is extremely hard.
And, because we’re talking about Walled Gardens, there is no universal identifier that readily connects an individual across the landscape. Not to mention, much of the provided sales data is not nearly as granular as necessary for probabilistic modeling.
But, is this as good as it gets?

A screenshot from the Measured website touting their integrations

A screenshot from the Kochava website touting their integrations
Method #2: Co-op Panel
Solutions like Circana (formerly NPD + IRI) have been a mainstay in retail advertising, specifically because most major retailers share sales data with them. They are able to enrich this data with additional metadata such as consumer demographics to allow for some semblance of cohorting. This has enabled a healthy brand / retailer relationship for decades.
These solutions provide detailed data and insights into consumer behavior, purchase patterns, brand performance, and help brands make better decisions about how their ad spend impacts consumer behavior. All of this is predicated on the fact that they can ‘close the loop’ with the retailers’ contributed sales data.
And, naturally, these solutions have been table stakes for large consumer advertisers for decades. They are unwieldy and expensive for smaller advertisers but just about everyone, at some scale, comes to rely on them.
The big risk: Many of the upcoming Walled Gardens are actually owned by the retailers (Retail Media Networks). Legacy retail brands such as Walmart or Target have historically shared data into these co-ops. But, as they launch digital advertising networks primarily predicated on their ability to close the measurement loop themselves, will they continue to contribute sales data to these co-ops?
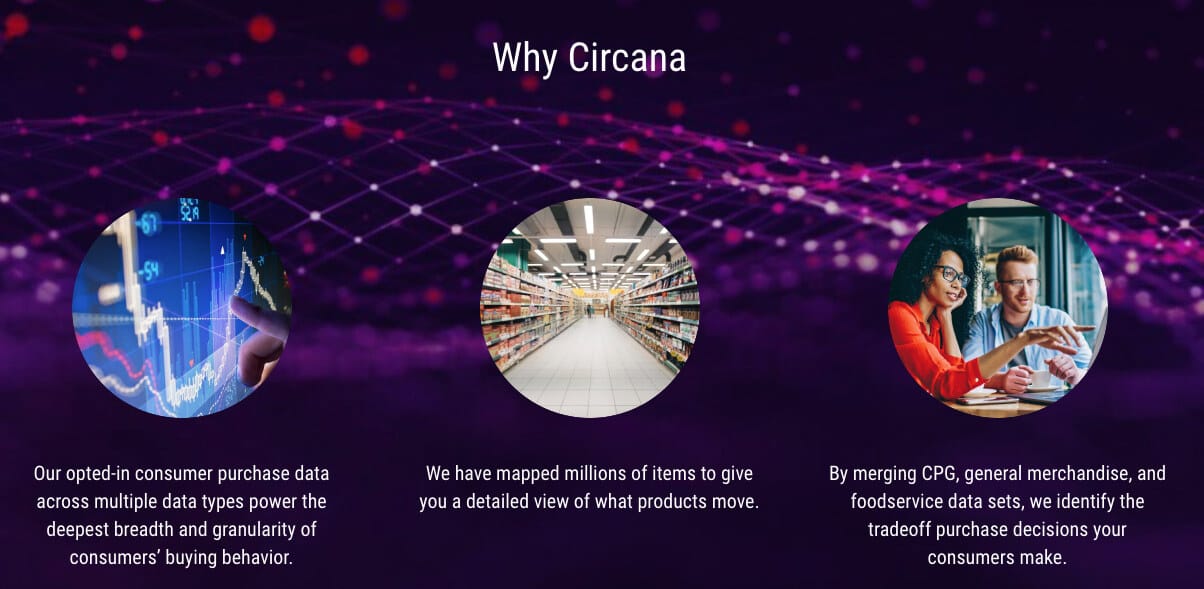
Method #3: Independent Panel
A third approach is for an independent measurement brand to establish a direct relationship with the consumer. Once done, they are capable of seeing sales data – who bought what. Ideally, these panels encourage consumers to submit personal data + purchases across all major retailers they buy from. The upside: they can see purchases across the spectrum and they have enough metadata to engage in specific cohorting — great for probabilistic attribution.
The challenge with this approach has always been scale and representativeness. Here are the questions any good marketing scientist will ask when confronted with an independent panel:
Are there enough consumers in your panel to gauge ROAS?
Are the type of consumers actually representative of the general population?
Can you get additional metadata to know these users?
Have you actually gotten them to submit purchases across their entire wallet, or just part of it?
In the digital age, scaling independent panels has become more possible than ever. Leading panels such as Fetch Rewards, Attain (fka Klover), and newly-public Ibotta have perfected the rewards-app-cum-consumer-panel playbook. Leveraging internet scale, they’ve managed to build multi-million consumer panels — something that would have seemed unthinkable just several years ago. That’s millions of consumers who are regularly submitting their purchases, whether by snapping a picture of their receipt, connecting their accounts, or otherwise.
With this scale, not only do these panels overcome the majority of the scale + representativeness questions, but also they offer fine-grained cohorting that represents a leap beyond the prior generation’s co-op panels.

Summing it all up…

From an ad platform’s perspective
It’s very important to give these large ad platforms due credit: yes, they are grading their own homework. But they’re also a step-change more effective than many of the advertising channels that existed before them. In fact, that’s the reason this practice has been largely accepted by brands in the first place.
And while Walled Gardens aren’t going anywhere fast, this problem is very real, as any CMO with a cocktail in hand in Cannes will confirm.
If ad platforms are able to develop better proxies for causation they’ll not only position themselves on the same team as the CMO, but also gain greater share.
Value-added Attribution Studies
Studies like the Facebook Nielsen Total Brand Effect with Lift, which bring in trusted third-party measurement partners, inspire confidence amongst brands, justifying past spend, in addition to encouraging future spend.
From an optics perspective, it appears to the CMO that the ad platform is putting its money where its mouth is.
Surveys on a sample of exposed users to gauge path to purchase — why did they buy it?
A/B Testing Brand Lift
Using control and holdout groups, measure the impact when a similar group doesn’t see the ads, how does it work.
Enable brands to run A/B tests where they turn off certain marketing channels, to assess incrementality.
Integration with 3P Measurement Partners
Providing the fodder for probabilistic attribution – metadata about users who see adds, their demographic & behavioral characteristics – will allow brands to better create cohorts of users and run attribution models.
Doing this in a privacy-safe way will be a challenge — but, hey, LiveRamp IDs are going strong on the open web, so it’s doable.
Again, to their credit, many ad platforms are already going down these paths. For example, leading ad platforms such as Facebook and Google have released open source Marketing mix Modeling software, which allows advertisers to do their own work to answer the question: which advertising is working vs. how much I am paying?
And, at the same time, we still have a long way to go before we reach the promised land…
Where do we go from here?
As always, the first step is admitting the reality: Brand marketers are panicked about over-attribution. With a blossoming of new Walled Garden ad networks all claiming credit for new sales, CMOs need a new toolkit to measure which half of their advertising works.
With privacy regulations + Walled Gardens, deterministic attribution across channels seems untenable anytime soon. And, long-trusted panel-based solutions may also come under threat depending on retailers’ decisions to contribute (or not!) to those co-ops. Independent panels seem promising, especially given the massive scale that the internet has unlocked — but it’s early days.
At the end of the day, the marketing world needs to get a few things right:
With all of these new advertising channels, we hope that it expands demand, rather than result in a zero sum game between ad channels.
Brands must have the ability to measure advertising spend + sales data if they’re expected to spend on advertising, especially on new, unproven channels.
The key is doing this across channels, not just within any given channel.
Support for probabilistic attribution methodologies is a must-have.
In a world in which the entire C-Suite – not just the CMO – is left wondering “which half of my advertising works?” this may just be the most important issue in marketing today.
Authors’ note: We don’t know everything :). Parts of this blog post are based on what we believe to be true today. Perhaps big ad platforms have already moved to a purely causation-based pricing model behind the scenes. If you feel that we’ve gotten any of the facts wrong, we’d love to hear from you.